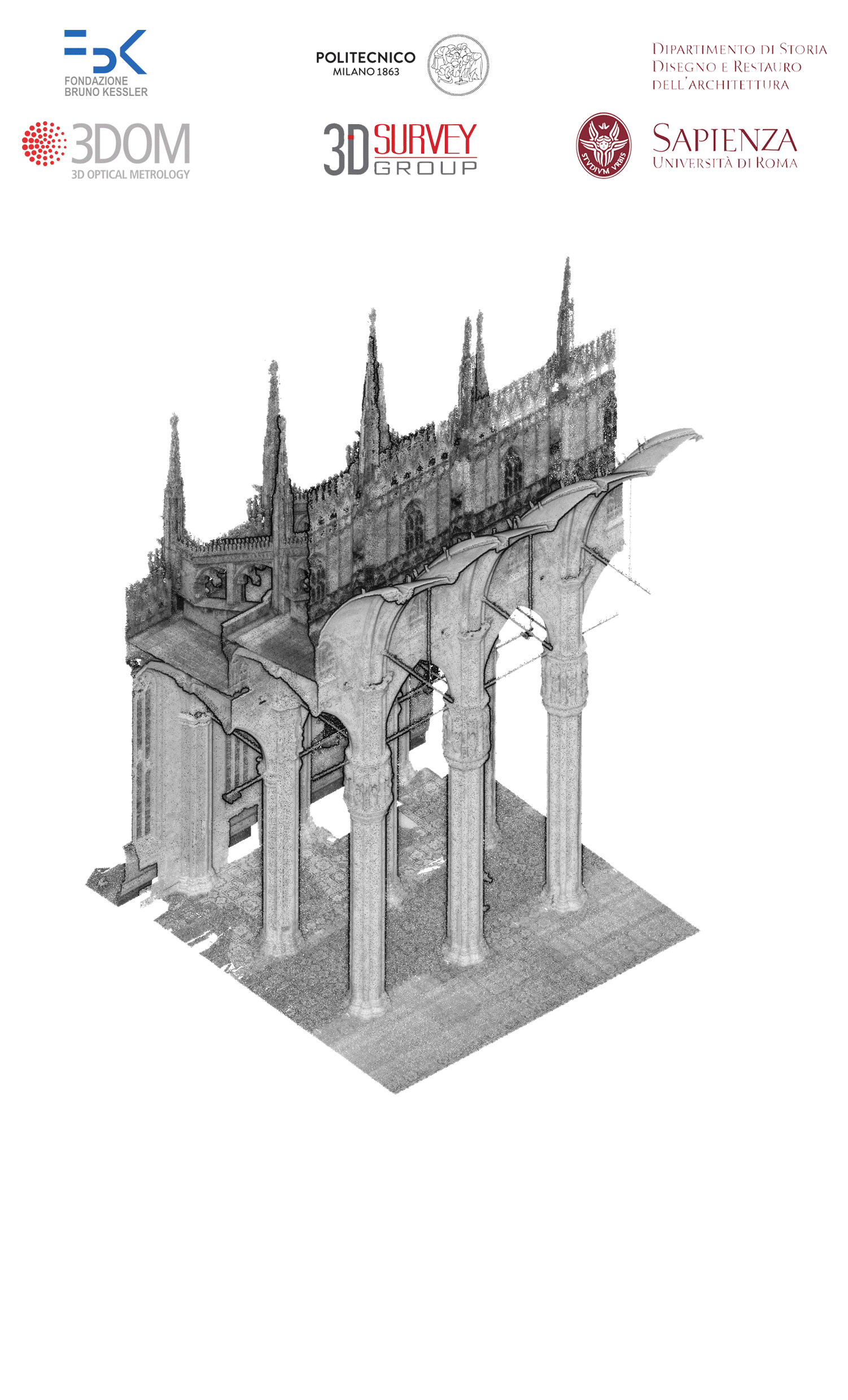
We present the first results of a new, rapid, and accurate Machine Learning method to semantically segment 3D heritage datasets based on a Multi-Layer Multi-Resolution (MLMC) approach. It deals with the introduction of point cloud classification methods that can play an essential role for better data usage, model definition, analysis and conservation in Cultural Heritage field.
The identification of precise architectural components in point clouds can be very useful because it allows the direct use of 3D point clouds for architectural interpretation inside and conservation activities planning, avoiding the modelling phase typical of HBIM (Historic Building Information Modelling). This latter phase is a time-consuming process which can also lead to a great simplification of detailed surfaces, losing the metric reliability intrinsic present in the acquired point clouds.
Teruggi et al., 2020
This research work is developed with 3DOMFBK and Università Sapienza di Roma.
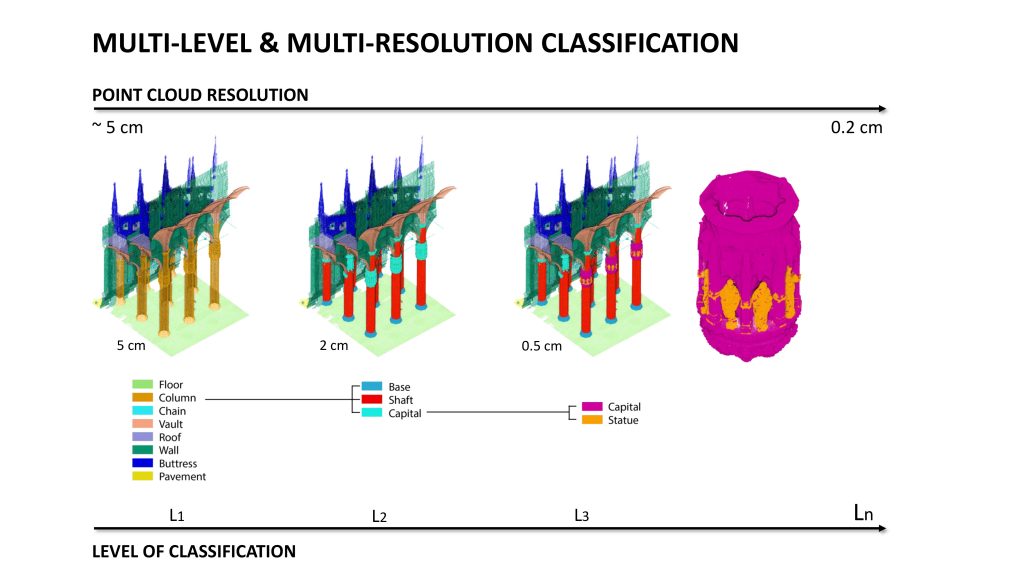
Read the full paper below:
- Teruggi S, Grilli E, Russo M, Fassi F, Remondino F. A Hierarchical Machine Learning Approach for Multi-Level and Multi-Resolution 3D Point Cloud Classification. Remote Sensing. Published online August 12, 2020:2598. doi:10.3390/rs12162598
Stay tuned for new updates!